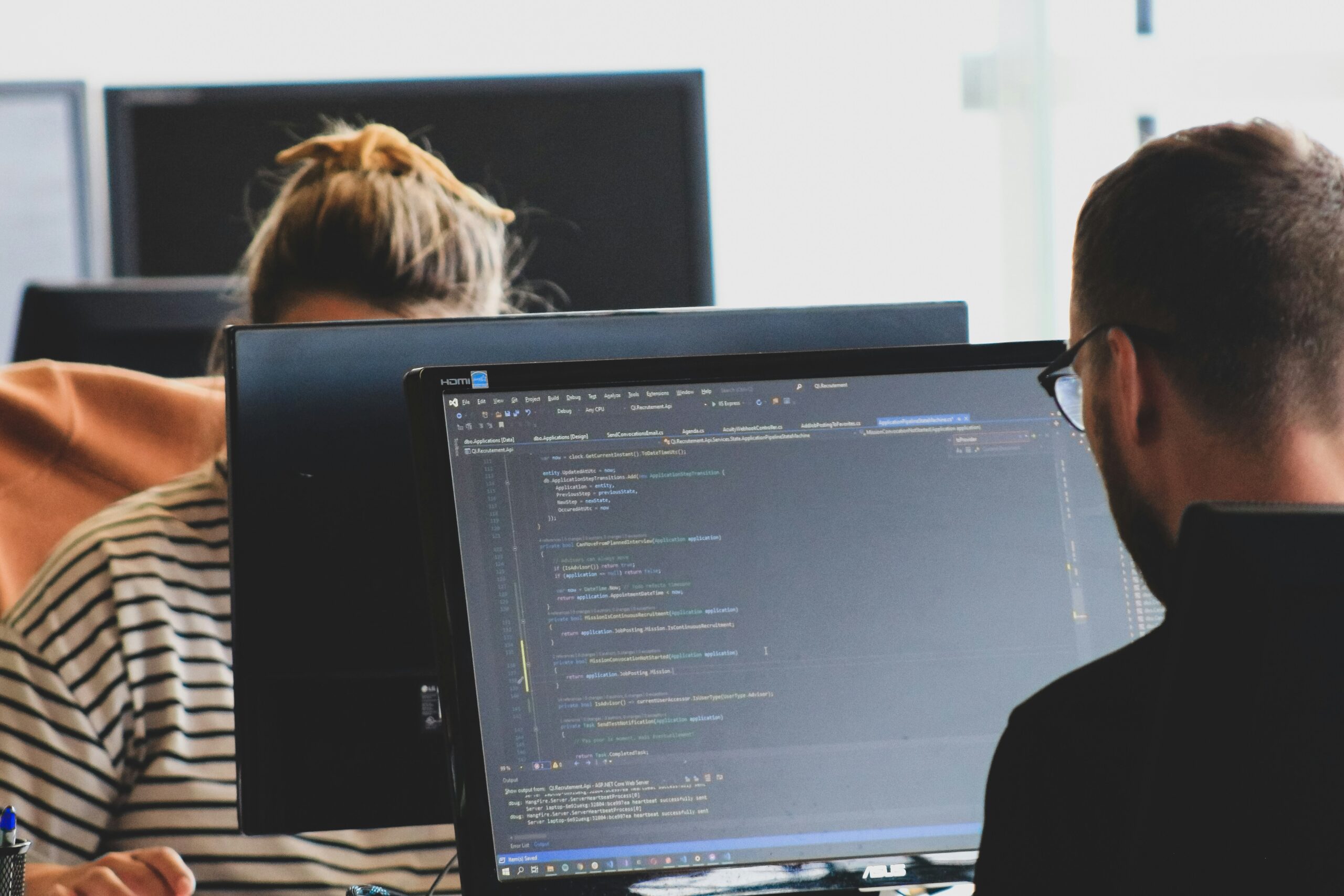
Introduction to AI Coding Assistants
As the demand for efficient software development continues to rise in the corporate tech landscape, organizations are increasingly turning to AI coding assistants. These sophisticated tools utilize artificial intelligence to support developers throughout various stages of coding, ultimately enhancing both productivity and efficiency. With the capacity to expedite mundane tasks, suggest code snippets, and identify errors, AI coding assistants are redefining the coding experience for professionals.
The burgeoning significance of these assistants can be attributed to the ever-growing complexities of software projects. In an environment where time is of the essence, the ability of AI coding tools to streamline coding processes becomes invaluable. By automating routine and repetitive tasks, these tools allow developers to focus on more critical aspects of software design and architecture. Their integration into daily workflows is proving to be an effective strategy for many teams seeking greater agility in their projects.
The growing reliance on AI in software development reflects a broader trend towards digital transformation. As organizations adapt to this shift, AI coding assistants are likely to play a central role in shaping the future of programming practices, offering a blend of speed, accuracy, and collaborative effectiveness that is essential for success in today’s fast-paced technological environment.
Criteria for Choosing an AI Coding Assistant
When selecting an AI coding assistant for corporate projects, several critical criteria should be taken into consideration to ensure the tool aligns with the unique needs of the development team. One of the foremost factors is integration capabilities. The chosen coding assistant should seamlessly integrate with existing tools and software already in use, such as version control systems, integrated development environments (IDEs), and project management applications. Enhanced compatibility minimizes disruption to workflows and allows teams to leverage the assistant effectively.
User-friendliness is another essential criterion; the tool should be intuitive and easy to navigate, ensuring that team members, regardless of their technical expertise, can utilize its features without extensive training. Complexity and a steep learning curve can hinder productivity, making it vital for organizations to choose an AI coding assistant that promotes a smooth onboarding experience.
Support for various programming languages is also a significant factor. A coding assistant that comprehensively covers multiple languages enhances its versatility, allowing teams to work on diverse projects without switching tools. This flexibility encourages collaboration and facilitates tackling various tasks efficiently.
Customization options should not be overlooked, as they allow teams to tailor the assistant to fit their specific workflows and preferences. An adaptable tool can significantly improve team productivity and satisfaction, making it easier to align the AI’s functionalities with the users’ requirements.
Lastly, cost considerations remain paramount. Organizations need to assess the pricing structure of different AI coding assistants, considering both upfront costs and potential long-term benefits. A tool that provides robust features and substantial ROI may justify a higher initial investment. Thus, aligning tool features with team needs, preferences, and budgets is crucial for the successful implementation of an AI coding assistant in corporate projects.
List of Top AI Coding Assistants for Corporate Use
1. GitHub Copilot – Specializing in code suggestion and auto-completion, GitHub Copilot uses machine learning models trained on billions of lines of code. It integrates seamlessly within popular coding environments like Visual Studio Code, providing contextual snippets and functions that enhance coding efficiency. This tool is particularly beneficial for developers looking to accelerate their coding processes and reduce mundane tasks associated with writing boilerplate code.
2. Kite – Kite is an AI-powered coding assistant that specializes in providing intelligent code completions while you type. It supports multiple programming languages, including Python, JavaScript, and Go. Key functionalities include line-of-code completions and documentation lookups. Kite’s capability to adapt to individual coding styles makes it a valuable asset for teams working on diverse corporate projects, facilitating smoother collaboration among team members.
3. Tabnine – Focused on predictive coding, Tabnine employs deep learning algorithms to predict and suggest code completions based on the developer’s existing work. By learning from your repository, Tabnine enhances productivity by speeding up the coding process. It supports a wide range of programming languages and integrates with most IDEs, making it a flexible choice for corporate environments needing customizable coding solutions.
4. Codeium – Offering features such as real-time code suggestions and natural language processing capabilities, Codeium aims to enhance the coding experience by allowing developers to write code using plain language queries. Its adaptability helps teams that require rapid iteration and prototyping across various languages. This makes Codeium a strong contender for corporate projects focused on innovation and agility.
5. DeepCode – DeepCode focuses on code review and quality assurance using AI techniques to provide feedback on existing code. It specializes in identifying bugs and vulnerabilities in real-time, thereby improving code quality before deployment. This tool is ideal for corporate teams aiming to maintain high coding standards and ensure secure software development practices.
Pros and Cons of Each AI Coding Assistant
In the rapidly evolving landscape of software development, AI coding assistants have emerged as valuable tools that can enhance productivity and streamline project workflows. However, understanding the advantages and disadvantages of each AI coding assistant is crucial for software teams to make informed decisions tailored to their specific project needs. Below, we evaluate the pros and cons of several popular AI coding assistants.
GitHub Copilot
Pros: GitHub Copilot integrates seamlessly with popular IDEs, providing real-time code suggestions that can significantly speed up the programming process. Its access to a vast array of libraries and frameworks makes it adaptable for various project types. Additionally, the familiarity of GitHub in the developer community boosts user confidence.
Cons: Although effective, GitHub Copilot may generate inaccurate or insecure code snippets, necessitating thorough reviews by developers. Additionally, its reliance on existing code can lead to reduced creativity, as it may discourage developers from experimenting with new solutions.
Kite
Pros: Kite offers an impressive feature set, including line-of-code completions and a built-in documentation tool that helps programmers obtain information without leaving their coding environment. Its lightweight nature ensures minimal disruption to the coding workflow.
Cons: Kite’s support may not extend to all programming languages, potentially limiting its applicability for teams using diverse stacks. Furthermore, its reliance on local machine resources can be a drawback for teams with lower-spec hardware.
Tabnine
Pros: Tabnine accommodates multiple programming languages and provides personalized code predictions based on the developer’s coding style. This adaptability makes it an ideal choice for teams working on varied projects.
Cons: While Tabnine enhances productivity, it can sometimes generate contextually irrelevant suggestions, leading to frustration. Additionally, the effectiveness of its predictions heavily depends on the quality of existing code within the project.
By weighing the strengths and weaknesses of each AI coding assistant, teams can more easily evaluate which tool aligns with their project specifications and goals.
Case Studies: Successful Corporate Implementations
In recent years, several corporations have embraced AI coding assistants to enhance their software development processes. Notable case studies illustrate how these tools can optimize workflows, mitigate challenges, and yield substantial outcomes. One such example is a leading financial institution that integrated an AI coding assistant into their application development life cycle. The aim was to reduce coding errors and accelerate project timelines. By employing tools like GitHub Copilot, the development team experienced a 30% decrease in coding errors, resulting in faster deployment times and improved software reliability.
Another success story comes from a technology startup that employed AI coding assistants to tackle high-pressure project demands. Faced with tight deadlines for a new client application, the developers leveraged an AI tool, which provided suggestions and auto-completions for code snippets. This allowed the team to maintain the pace of development, ultimately delivering the project two weeks ahead of schedule, much to the satisfaction of their client. The immediate outcome was a boost in client trust and future partnerships, showcasing the swift adaptability of AI in real-world applications.
A large retail chain also turned to AI coding assistants to streamline their backend systems for better inventory management. By utilizing custom machine learning algorithms integrated into AI tools, the developers not only improved system efficiency but also enabled predictive analytics for stock levels. This transition, while initially met with concerns over employee roles, ultimately resulted in increased productivity and allowed the team to focus on more strategic initiatives rather than routine coding tasks.
These case studies emphasize that implementing AI coding assistants can serve as a vital asset for organizations, facilitating improved efficiency, reduced errors, and enhanced project outcomes. The experience of these corporations provides valuable insights for others considering similar integrations into their development workflows.
Comparative Summary of AI Coding Assistants
As the demand for efficient coding solutions continues to grow in corporate environments, a variety of AI coding assistants have emerged to meet the needs of software developers. Below is a comparative summary that highlights the key features, pricing models, support options, and unique selling points of the top AI coding assistants currently available.
AI Coding Assistant | Key Features | Pricing | Support | Unique Selling Points |
---|---|---|---|---|
GitHub Copilot | Code suggestions, multi-language support, and context-aware completions. | $10/month or $100/year | Email and community forums. | Deep integration with Visual Studio Code and GitHub platforms. |
Tabnine | AI-driven code completion, supports many languages, and customizable models. | Free tier; Pro at $12/month. | 24/7 support for Pro users. | Personal AI models allow customization to project-specific needs. |
Kite | Code completions, function signatures, and code documentation. | Free version available; Pro at $19.90/month. | Email support and user community. | Fast and lightweight performance with over 30 language support. |
Codex | Natural language to code, full-function completion, and API integration. | Varies by usage; pay-per-call model. | Online resources and documentation. | Ability to convert natural language commands into code swiftly. |
This table serves as an efficient reference for evaluating the various AI coding assistants, incorporating fundamental aspects that can significantly impact their utility in corporate projects. By understanding each assistant’s features and capabilities, organizations can make informed decisions that align with their software development needs.
Challenges and Limitations of AI Coding Assistants
While AI coding assistants offer numerous benefits to organizations, several challenges and limitations must be addressed. One significant issue is the lack of human intuition that these tools possess. Although AI can analyze patterns and generate code based on existing data, it inherently lacks the understanding of context that a human developer brings to the table. This deficiency can lead to suboptimal solutions or misunderstandings of project requirements, especially in complex coding scenarios where nuanced decisions are necessary.
Another critical challenge is the dependency on training data. AI coding assistants learn from vast datasets that may contain biases or outdated practices. This reliance on historical data can inadvertently perpetuate inaccuracies, thus creating a risk of producing code that does not align with current industry standards or best practices. Additionally, organizations may find it challenging to ensure that the training datasets for these AI tools are relevant, comprehensive, and updated regularly to reflect the evolving nature of programming languages and frameworks.
Furthermore, the necessity of monitoring AI outputs for quality control cannot be overstated. While AI may assist in automating repetitive coding tasks, its outputs are not infallible. Developers must review and validate the code generated by these tools to ensure accuracy and efficacy. This oversight can introduce an additional layer of workload for teams already pressed for time, potentially negating some of the efficiency gains that AI is designed to provide. Establishing a robust feedback mechanism is essential to improve the performance of AI coding assistants and to seamlessly integrate them into the corporate workflow.
In conclusion, while AI coding assistants have the potential to enhance productivity and streamline workflows, organizations must navigate several significant challenges and limitations to fully leverage their capabilities. Ensuring the right balance between automation and human oversight is essential for optimizing the benefits of these advanced tools.
Future of AI in Coding Assistance
The future of AI in coding assistance holds significant promise, particularly within corporate environments where efficiency and productivity are paramount. As artificial intelligence continues to evolve, its applications in software development are poised to expand, effectively transforming traditional coding workflows. One notable trend is the increasing integration of machine learning algorithms that can analyze vast amounts of code and learn from patterns, significantly streamlining the coding process.
In addition, advancements in natural language processing (NLP) are expected to enhance the interaction between developers and AI tools. As these coding assistants evolve, they will likely become more adept at translating human intentions into functional code snippets, thereby reducing the time developers spend on routine tasks. This level of automation will not only alleviate the coding burden but also enable developers to focus on more complex and creative aspects of software development, which is vital for corporate innovation.
Moreover, as remote work becomes more prevalent, AI coding assistants are expected to evolve to support collaborative efforts among distributed teams. Features such as real-time code suggestions, automatic error detection, and seamless integration with existing project management tools will become core components of these platforms. This paradigm shift will facilitate enhanced cooperation among team members, leading to improved output quality and accelerated project timelines.
Looking ahead, industries may witness the emergence of specialized AI assistants tailored to specific sectors, like finance, healthcare, or automotive. Such tools will be designed to integrate regulatory compliance and sector-specific best practices into the coding process, providing companies with a robust framework to maintain quality and security standards. Overall, the forward trajectory of AI coding assistants suggests a future where coding is not just automated but is also a collaborative effort driven by intelligent technology, empowering businesses to meet the growing demands of the digital landscape.
Conclusion and Recommendations
In the evolving landscape of software development, AI coding assistants have emerged as valuable tools that can significantly enhance productivity and streamline workflows for corporate projects. This blog post has examined various AI coding assistants, highlighting their advantages and disadvantages. The insights gained suggest that while these tools offer substantial benefits—such as improved code quality, increased efficiency, and predictive capabilities—they also come with challenges like dependency risks and potential limitations in understanding complex coding requirements.
For organizations contemplating the integration of AI coding assistants into their development processes, a careful evaluation is crucial. It is recommended that companies consider their specific needs and workflows to select the most appropriate tool. This includes assessing the AI’s accuracy in matching the company’s coding standards and its adaptability to the technologies in use. Organizations should also encourage developer training to ensure that team members are equipped to leverage these tools effectively and to mitigate any risks associated with overreliance on automation.
Furthermore, it is advisable for companies to pilot AI coding assistants in smaller project scopes before full-scale implementation. This approach enables the teams to gauge the tool’s impact on productivity and identify any necessary adjustments. Additionally, maintaining a balance between automated assistance and human oversight will help preserve coding integrity, ensuring that the final output meets the expected standards. Investing in AI coding assistants can yield significant long-term benefits, provided that the selection process aligns with the organization’s goals and objectives.
In conclusion, AI coding assistants represent a transformative technology in the domain of software development. By fostering collaboration between developers and these sophisticated tools, organizations can thrive in an increasingly competitive environment while maximizing their project outcomes.